AIhub coffee corner: Bad practice in the publication world
The AIhub coffee corner captures the musings of AI experts over a short conversation. This month we tackle the topic of bad practice in the sphere of publication. Joining the conversation this time are: Sanmay Das (Virginia Tech), Tom Dietterich (Oregon State University), Sabine Hauert (University of Bristol), and Sarit Kraus (Bar-Ilan University). Sabine Hauert: […]
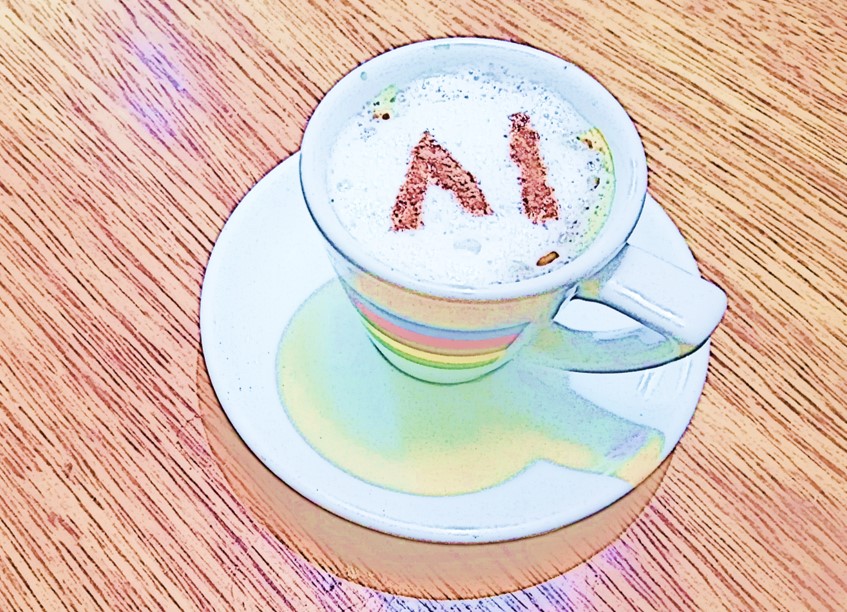
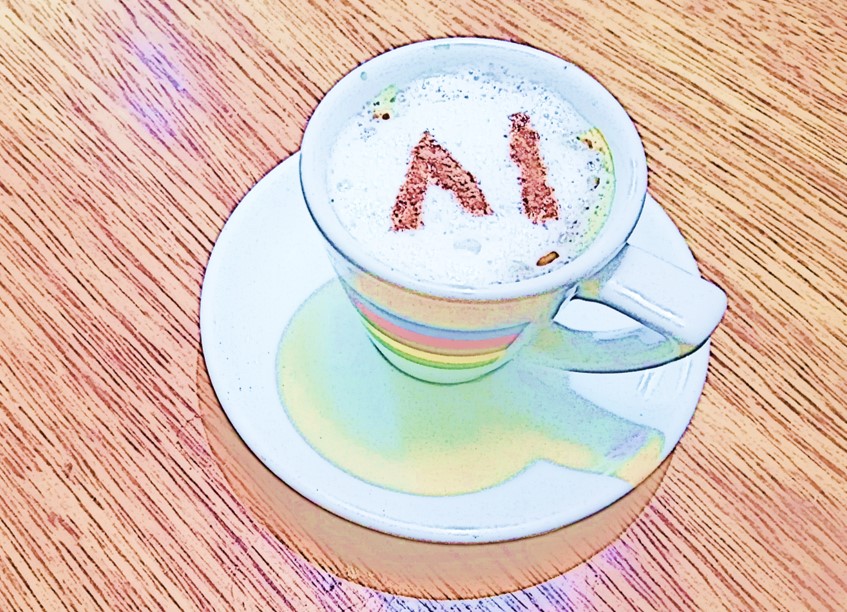
The AIhub coffee corner captures the musings of AI experts over a short conversation. This month we tackle the topic of bad practice in the sphere of publication. Joining the conversation this time are: Sanmay Das (Virginia Tech), Tom Dietterich (Oregon State University), Sabine Hauert (University of Bristol), and Sarit Kraus (Bar-Ilan University).
Sabine Hauert: Today’s topic is bad practice in the publication world. For example, people trying to cheat the review system, paper mills. What bad behaviors have you seen, and is it really a problem?
Tom Dietterich: Well, I can talk about it from an arXiv point of view. The main bad behavior we see is citation stuffing. We’re seeing authors writing very low-quality papers that cite a bunch of references that are not really relevant to the paper. It seems that their goal is to have a paper that is good enough for arXiv to release, but they don’t want anybody to actually read it. They just want Google Scholar to index the citations. And we’ve seen it in various forms. The typical papers are things where they claim to be doing, say, heart disease using machine learning, but they’re just downloading the Cleveland heart dataset from the UCI repository, running standard machine learning algorithms on it, and writing up the results. They often have a very beautiful introduction, presumably written by ChatGPT, all about heart disease and its causes. But then, the references are irrelevant.
I’ve seen other ones where it seemed like it was more or less a real paper, but for every citation they made that was relevant, they would have another one that was irrelevant. So, they all came in pairs. That’s the main thing we see, basically trying to boost citation counts by having things released on arXiv.
Sabine: And it does matter, this citation stuffing. I recently heard of a panel for faculty appointments. They screened a ton of applications and the ones that made it to the interview had great things going for them – they worked in interesting research areas, they had high h-indices, they were very productive. However, when the panel spoke to them, they realized that they had never worked with anyone in the field that they claimed to work in, it was just purely a niche they’d invented. They were very productive in gaming the system. Without talking to that person, they made it to the top of the pile. Unfortunately, that does remove a lot of people at the screening stage who didn’t play that game, so it really does matter that they’re doing that. Are there other examples of bad behavior that you’ve seen?
Sarit Kraus: One example is sending the same paper to multiple conferences. Sometimes people will make small changes but usually they don’t even try to make the papers different. Another problem is poor quality reviews. A lot of reviewers are using LLMs to write the review for them, but then they don’t read the output before submitting the review. I don’t mind the reviewers using a LLM to generate a review, as long as they read it and check the paper to see if it is reasonable.
Tom: One of my colleagues put jailbreak instructions in white text to ChatGPT into a homework assignment to find out how many students were using LLMs to answer the homework and were not reading the results. We could do that with every conference paper – embed something that encourages the LLM to output a certain phrase that we could then scan for. Basically, fingerprint the output.
Sabine: How big of a problem is bad practice? Is it 1% of submissions that are bad practice or is it something that’s really growing?
Tom: Of course, we only know the ones that we detect, but on arXiv it’s much less than 1%. In the machine learning category, we probably have 15,000 papers released under machine learning and maybe we found 5-10 with problems. But we don’t have any measure of our missed alarms.
Sabine: Should we do anything about it?
Tom: Well, the biggest problem I think has been the reviewing rings at conferences. I haven’t seen any of the evidence, but there are reports from ICML and NeurIPS. The big problem there is the bidding system for reviewers to bid on papers they want to review. That basically opens up people bidding on each other’s papers. We have to change how we assign reviewers to papers to break those rings up. We also lack an enforcement mechanism for punishing people who break the rules. The conferences don’t have legal staff, they don’t have all the processes in place to collect the evidence and be able to present it, and no one has the time to spend contacting the supervisors. What are the penalties that should be applied? What kind of due process should we have? The community needs to decide these things.
Sabine: It’s also a fine line because, in a small area of work you might bid for people’s papers that you know well because you happen to be in a small community. That might be a legitimate set of papers that you want to review, and you review them in an accurate way.
Sarit: Bidding may not be a good mechanism. At IJCAI, we utilize keywords and similar methods to assign papers to reviewers. However, we still have bidding, and I don’t think this bidding is a good idea. However, I think that even if we remove the bidding system, we will still have a big problem. And the problem is, as Tom said, the lack of punishment. There is no punishment for fabricating, for cheating, nothing. It was good when we were a small community, and it was enough of a punishment for the rest of the community to know that somebody broke the rules. If there was some entity that could take care of these events, that would be very useful. We are worried because if we put a name in the public domain, they can sue us, because we don’t have the legal backing. And we want to spend our time doing our research. We are volunteers, managing conferences, and once we finish organizing one, we want some quiet for a few years.
Sabine: Could part of this bad practice just be not knowing better, not knowing that this is not the way it’s done? Could more education help?
Sarit: No, it’s not education. People know that they are breaking the rules.
Tom: I mean, they’re exchanging paper titles with each other so they know who has authored which papers.
Sabine: Yes, that’s explicit.
Sarit: The benefit of cheating is much higher than the risk of being caught because you are not getting punished. If your paper is not accepted at the current conference, you can just submit it to another conference next year.
Tom: There is this joint body, the Artificial Intelligence Scientific Organizations Coordinating Council (AISOCC), that AAAI has been helping to establish. It is trying to get people from many conferences to talk to each other about these kinds of issues. I think OpenReview, which supports the review process for most of the machine learning conferences, has information that they can pool across conferences. NeurIPS recently revised their Code of Conduct to explicitly allow information sharing between sister organizations.
My thought is that someplace like OpenReview could be the group that, if the various users pool funds, they can hire a legal team and establish a set of standards and terminology that could then be adopted by all the various conferences. And they could be the center of the enforcement mechanism as well.
Sabine: At the least, putting a little star next to papers suspected of bad practice.
Tom: I think that the goal then would be to go beyond that and actually publicize the rule breakers. Contact their management, get them fired.
Sanmay Das: I feel like conferences should be doing some coordination to at least have a common code of conduct, and we probably need legal advice to make sure that it goes the right way. I think the one thing that needs to be done is that, when people submit to a conference, they commit to a process which will be followed by the conference. And if they are blacklisted by that process, they’ve agreed to that as a condition of submission.
The other thing I have to point out though, is that there have been situations that I’ve been aware of where very senior people in the community are pretty clearly engaging in these kinds of practices. And they can defend themselves very strongly. And it’s hard to actually fully pin it down. They can claim that they didn’t do any of this intentionally. Nobody wants to get involved in a legal fight about this.
Sabine: I do wonder when people submit, whether they’re going to read all those rules [regarding LLM use, for example] when they click. However, if there was a short step where they were questioned on whether they’d engaged in a particular bad practice, it might make them feel just bad enough so they don’t engage in it, or just make requirements explicit. I like to be hopeful about people.
Sarit: Before IJCAI 2019, I was like you, you know “why will people cheat? We are all involved in research, that’s our life, why would a person cheat?” But then, I encountered a case where someone managed to review their own paper. When confronted, they claimed it was their student who had submitted it. What could I do? I rejected all of that person’s submissions for that conference, but I had no way to carry this information forward to the next event. Who knows if they repeated the behavior at the next conference? I stopped being naïve at that point.